Attend an event
Connect with our local innovation ecosystem
Join the action at one of our many campus events that are open to the public. Foster new connections, cultivate new ideas, and open the door to new opportunities.
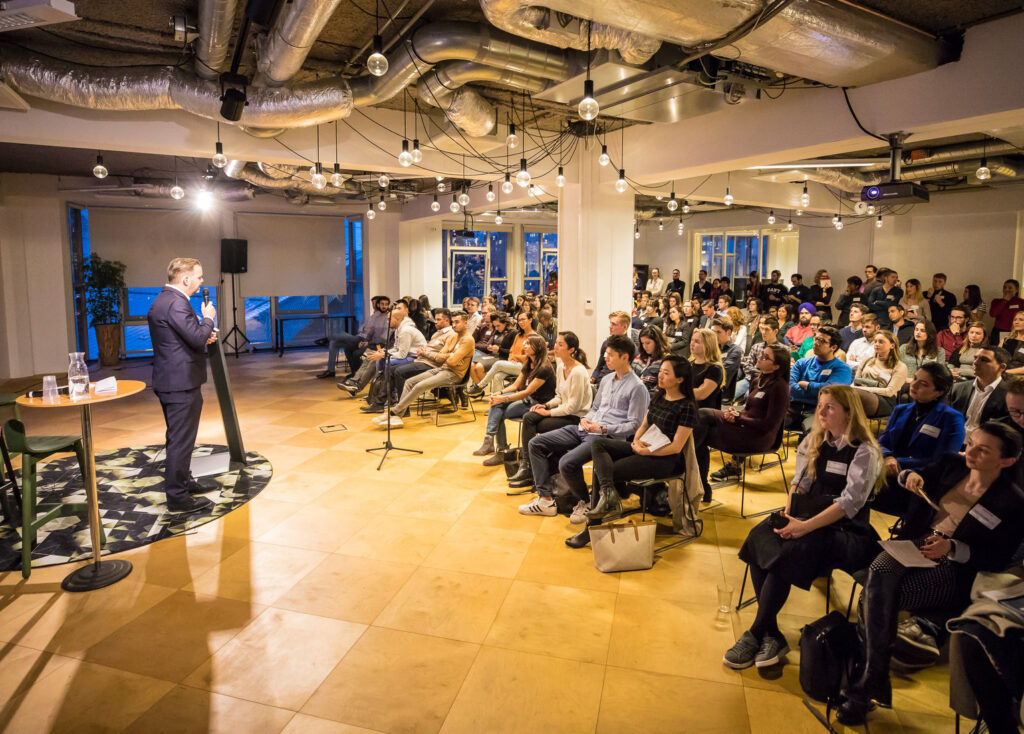
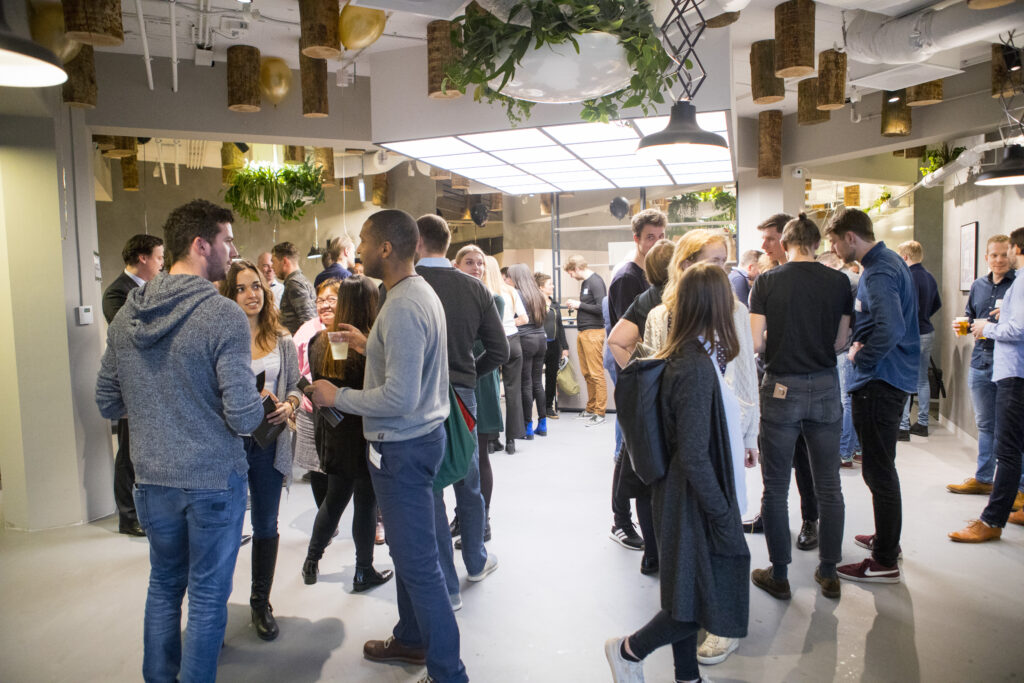
Attend an event
Join the action at one of our many campus events that are open to the public. Foster new connections, cultivate new ideas, and open the door to new opportunities.